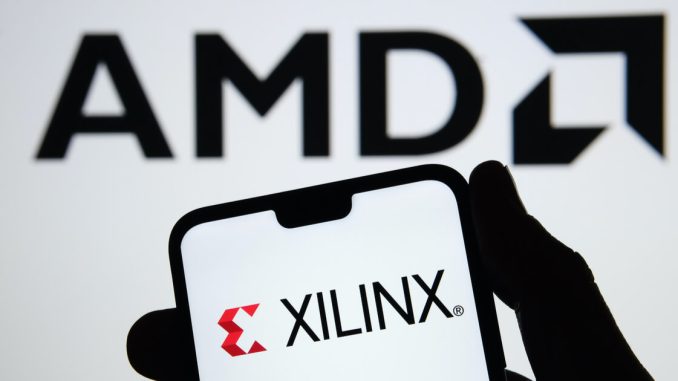
Caroline Bishop
Jan 09, 2025 03:07
AMD introduces optimizations for Visual Language Models, enhancing speed and accuracy in diverse applications like medical imaging and retail analytics.
Advanced Micro Devices (AMD) has announced significant enhancements to Visual Language Models (VLMs), focusing on improving the speed and accuracy of these models across various applications, as reported by the company’s AI Group. VLMs integrate visual and textual data interpretation, proving essential in sectors ranging from medical imaging to retail analytics.
Optimization Techniques for Enhanced Performance
AMD’s approach involves several key optimization techniques. The use of mixed-precision training and parallel processing allows VLMs to merge visual and text data more efficiently. This improvement enables faster and more precise data handling, which is crucial in industries that demand high accuracy and quick response times.
One notable technique is holistic pretraining, which trains models on both image and text data concurrently. This method builds stronger connections between modalities, leading to better accuracy and flexibility. AMD’s pretraining pipeline accelerates this process, making it accessible for clients lacking extensive resources for large-scale model training.
Improving Model Adaptability
Instruction tuning is another enhancement, allowing models to follow specific prompts accurately. This is particularly beneficial for targeted applications such as tracking customer behavior in retail settings. AMD’s instruction tuning improves the precision of models in these scenarios, providing clients with tailored insights.
In-context learning, a real-time adaptability feature, enables models to adjust responses based on input prompts without further fine-tuning. This flexibility is advantageous in structured applications like inventory management, where models can quickly categorize items based on specific criteria.
Addressing Limitations in Visual Language Models
Traditional VLMs often struggle with sequential image processing or video analysis. AMD addresses these limitations by optimizing VLM performance on its hardware, facilitating smoother sequential input handling. This advancement is critical for applications requiring contextual understanding over time, such as monitoring disease progression in medical imaging.
Enhancements in Video Analysis
AMD’s improvements extend to video content understanding, a challenging area for standard VLMs. By streamlining processing, AMD enables models to efficiently handle video data, providing rapid identification and summarization of key events. This capability is particularly useful in security applications, where it reduces the time spent analyzing extensive footage.
Full-Stack Solutions for AI Workloads
AMD Instinct™ GPUs and the open-source AMD ROCm™ software stack form the backbone of these advancements, supporting a wide range of AI workloads from edge devices to data centers. ROCm’s compatibility with major machine learning frameworks enhances the deployment and customization of VLMs, fostering continuous innovation and adaptability.
Through advanced techniques like quantization and mixed-precision training, AMD reduces model size and speeds up processing, cutting training times significantly. These capabilities make AMD’s solutions suitable for diverse performance needs, from autonomous driving to offline image generation.
For additional insights, explore the resources on Vision-Text Dual Encoding and LLaMA3.2 Vision available through the AMD Community.
Image source: Shutterstock
Be the first to comment